Human features in neural network chess learning: Study
By ANI | Published: November 15, 2022 05:31 PM2022-11-15T17:31:09+5:302022-11-15T23:05:07+5:30
Published in the journal 'Proceedings of the National Academy of Sciences (PNAS) on November 14, a study reveals that ...
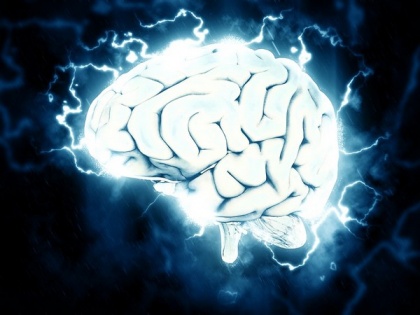
Human features in neural network chess learning: Study
Published in the journal 'Proceedings of the National Academy of Sciences (PNAS) on November 14, a study reveals that the neural network engine AlphaZero learns chess by playing against itself and reliably wins games against top human chess players.
According to a team of researchers, the neural network interpretability is a research area that encompasses a wide range of approaches and challenges. Our interest in relating network activations to human concepts means we focus primarily on so-called concept-based explanations.
Concept-based methods use human-understandable concepts to explain neural network decisions instead of using input features (e.g., pixels). As seen in refs, concept-based explanations have been successfully used in complex scientific and medical domains. 20-27 and in concurrent work on an agent trained to play the board game Hex, as well as in studying Go-playing agents using concepts derived from natural language annotations. Similar to many input feature-based methods, concept methods also only represent correlation and not causation. While there exist causal concept-based methods, this work focuses on analysis without assuming a causal graph that could incorrectly represent the internal mechanism.
A key step for these methods is to operationalize human-understandable concepts. One common approach, for example, is to define a concept by a set of exemplars. In the case of chess, however, we do not need to start from scratch; many standard concepts (e.g., king safety or material advantage) are already expressed in simplified, easy-to-compute functions inside modern chess engines.
An analysis revealed that although AlphaZero has never observed a human game of chess, many characteristics of human chess players were discernible throughout AlphaZero's training. Analysis of the neural network's opening strategy found that AlphaZero rapidly narrowed down possibilities from an initial range of possible moves. According to the authors, the findings suggest that AlphaZero is capable of relatively rapid skill learning over an extended training period, according to the authors.
( With inputs from ANI )
Disclaimer: This post has been auto-published from an agency feed without any modifications to the text and has not been reviewed by an editor
Open in app